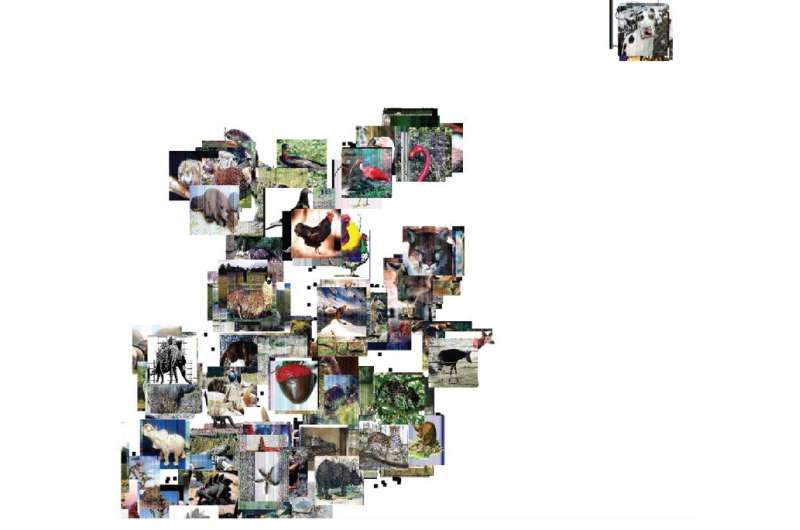
Figuring out structure-property family members is a key objective of fabrics analysis, in line with Joshua Agar, a college member in Lehigh College’s Division of Fabrics Science and Engineering. And but lately no metric exists to grasp the constitution of fabrics on account of the complexity and multidimensional nature of constitution.
Synthetic neural networks, a kind of gadget finding out, may also be skilled to spot similarities―or even correlate parameters equivalent to constitution and houses―however there are two main demanding situations, says Agar. One is that almost all of huge quantities of knowledge generated via supplies experiments are by no means analyzed. That is in large part as a result of such pictures, produced via scientists in laboratories everywhere the arena, are infrequently saved in a usable approach and now not most often shared with different analysis groups. The second one problem is that neural networks aren’t very efficient at finding out symmetry and periodicity (how periodic a subject material’s constitution is), two options of maximum significance to supplies researchers.
Now, a workforce led via Lehigh College has advanced a unique gadget finding out way that may create similarity projections by means of gadget finding out, enabling researchers to look an unstructured symbol database for the primary time and determine traits. Agar and his collaborators advanced and skilled a neural community fashion to incorporate symmetry-aware options after which implemented their way to a suite of 25,133 piezoresponse pressure microscopy pictures amassed on various supplies methods over 5 years on the College of California, Berkeley. The effects: they have been ready to crew equivalent categories of subject material in combination and apply traits, forming a foundation during which to begin to perceive structure-property relationships.
“One of the most novelties of our paintings is that we constructed a unique neural community to grasp symmetry and we use that as a function extractor to make it significantly better at figuring out pictures,” says Agar, a lead creator of the paper the place the paintings is described: “Symmetry-Conscious Recursive Symbol Similarity Exploration for Fabrics Microscopy,” printed nowadays in npj Computational Fabrics. Along with Agar, authors come with, from Lehigh College: Tri N. M. Nguyen, Yichen Guo, Shuyu Qin and Kylie S. Frew and, from Stanford College: Ruijuan Xu. Nguyen, a lead creator, used to be an undergraduate at Lehigh College and is now pursuing a Ph.D. at Stanford.
The workforce used to be ready to reach at projections via using Uniform Manifold Approximation and Projection (UMAP), a non-linear dimensionality aid methodology. This way, says Agar, permits researchers to be informed “…in a fuzzy approach, the topology and the higher-level constitution of the knowledge and compress it down into 2D.”
“When you educate a neural community, the result’s a vector, or a suite of numbers that may be a compact descriptor of the options. The ones options lend a hand classify issues in order that some similarity is discovered,” says Agar. “What is produced continues to be relatively massive in house, despite the fact that, as a result of you could have 512 or extra other options. So, then you need to compress it into an area {that a} human can comprehend equivalent to 2D, or three-D―or, possibly, 4D.”
By way of doing this, Agar and his workforce have been ready to take the 25,000-plus pictures and crew very equivalent categories of subject material in combination.
“Identical varieties of constructions in subject material are semantically shut in combination and likewise positive traits may also be seen in particular when you practice some metadata filters,” says Agar. “When you get started filtering via who did the deposition, who made the fabric, what have been they looking to do, what’s the subject material machine… you’ll be able to truly begin to refine and get increasingly similarity. That similarity can then be related to different parameters like houses.”
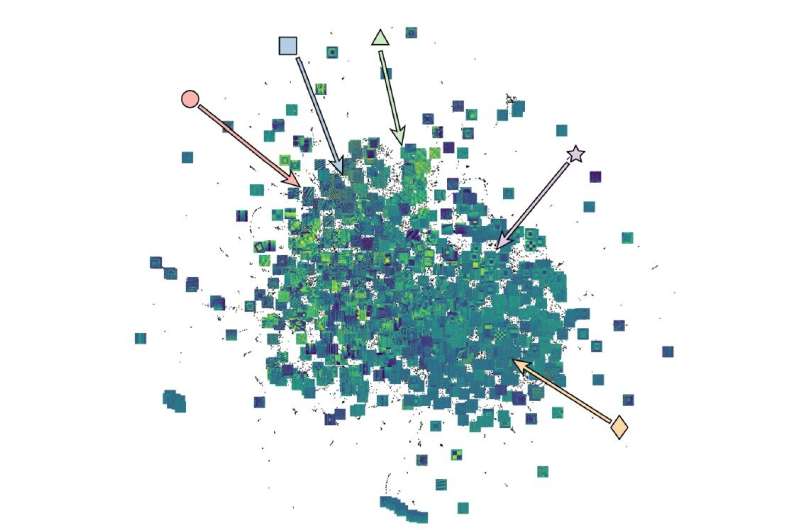
This paintings demonstrates how stepped forward records garage and control may all of a sudden boost up supplies discoveries. In step with Agar, of explicit worth are pictures and knowledge generated via failed experiments.
“No person publishes failed effects and that is the reason a large loss as a result of then a couple of years later anyone repeats the similar line of experiments,” says Agar. “So, you waste truly just right assets on an experiment that most probably may not paintings.”
As a substitute of shedding all of that data, the knowledge that has already been amassed might be used to generate new traits that experience now not been observed ahead of and pace discovery exponentially, says Agar.
This find out about is the primary “use case” of an cutting edge new data-storage undertaking housed at Oak Ridge Nationwide Laboratory referred to as DataFed. DataFed, in line with its site is “…a federated, big-data garage, collaboration, and full-life-cycle control machine for computational science and/or records analytics inside dispensed high-performance computing (HPC) and/or cloud-computing environments.”
“My workforce at Lehigh has been a part of the design and building of DataFed in the case of making it related for medical use instances,” says Agar. “Lehigh is the primary are living implementation of this fully-scalable machine. It is a federated database so someone can pop up their very own server and be tied to the central facility.”
Agar is the gadget finding out skilled on Lehigh College’s Presidential Nano-Human Interface Initiative workforce. The interdisciplinary initiative, integrating the social sciences and engineering, seeks to grow to be the ways in which people engage with tools of medical discovery to boost up inventions.
“One of the most key targets of Lehigh’s Nano/Human Interface Initiative is to position related data on the fingertips of experimentalists to offer actionable data that permits extra knowledgeable decision-making and speeds up medical discovery,” says Agar. “People have restricted capability for reminiscence and recollection. DataFed is a modern day Memex; it supplies a reminiscence of medical data that may simply be discovered and recalled.”
DataFed supplies an extremely tough and valuable instrument for researchers engaged in interdisciplinary workforce science, permitting researchers who’re participating on workforce tasks situated in numerous/far off places to get entry to each and every different’s uncooked records. This is likely one of the key elements of our Lehigh Presidential Nano/Human Interface (NHI) Initiative for accelerating medical discovery,” says Martin P. Harmer, Alcoa Basis Professor in Lehigh’s Division of Fabrics Science and Engineering and Director of the Nano/Human Interface Initiative.
Additional info:
Symmetry-aware recursive symbol similarity exploration for supplies microscopy, npj Computational Fabrics, DOI: 10.1038/s41524-021-00637-y
Quotation:
A singular neural community to grasp symmetry, pace supplies analysis (2021, October 8)
retrieved 12 March 2023
from https://phys.org/information/2021-10-neural-network-symmetry-materials.html
This report is matter to copyright. Aside from any honest dealing for the aim of personal find out about or analysis, no
section is also reproduced with out the written permission. The content material is equipped for info functions best.
Supply By way of https://phys.org/information/2021-10-neural-network-symmetry-materials.html