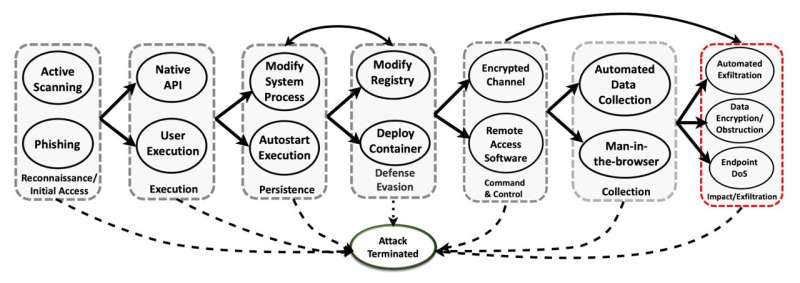
Scientists have taken a key step towards harnessing a type of synthetic intelligence referred to as deep reinforcement studying, or DRL, to offer protection to laptop networks.
When confronted with refined cyberattacks in a rigorous simulation surroundings, deep reinforcement studying used to be efficient at preventing adversaries from achieving their objectives as much as 95 % of the time. The end result provides promise for a task for independent AI in proactive cyber protection.
Scientists from the Division of Power’s Pacific Northwest Nationwide Laboratory documented their findings in a analysis paper and introduced their paintings Feb. 14 at a workshop on AI for Cybersecurity all the way through the once a year assembly of the Affiliation for the Development of Synthetic Intelligence in Washington, D.C.
The start line used to be the advance of a simulation atmosphere to check multistage assault eventualities involving distinct varieties of adversaries. Advent of this kind of dynamic attack-defense simulation atmosphere for experimentation itself is a win. The surroundings provides researchers a technique to evaluate the effectiveness of various AI-based defensive strategies underneath managed check settings.
Such equipment are crucial for comparing the efficiency of deep reinforcement studying algorithms. The process is rising as a formidable decision-support software for cybersecurity professionals—a protection agent being able to be informed, adapt to briefly converting instances, and make selections autonomously. Whilst different types of AI are same old to hit upon intrusions or filter out unsolicited mail messages, deep reinforcement studying expands defenders’ skills to orchestrate sequential decision-making plans of their day by day face-off with adversaries.
Deep reinforcement studying provides smarter cybersecurity, the facility to hit upon adjustments within the cyber panorama previous, and the chance to take preemptive steps to scuttle a cyberattack.
DRL: Selections in a extensive assault area
“An efficient AI agent for cybersecurity must sense, understand, act and adapt, in response to the guidelines it may possibly accumulate and on the result of selections that it enacts,” stated Samrat Chatterjee, a knowledge scientist who introduced the group’s paintings. “Deep reinforcement studying holds nice possible on this area, the place the selection of gadget states and motion alternatives will also be massive.”
DRL, which mixes reinforcement studying and deep studying, is particularly adept in scenarios the place a chain of selections in a posh atmosphere want to be made. Just right selections resulting in fascinating effects are strengthened with a favorable praise (expressed as a numeric price); dangerous alternatives resulting in unwanted results are discouraged by the use of a detrimental value.
It is very similar to how other people be informed many duties. A kid who does their chores may obtain sure reinforcement with a desired playdate; a kid who does not do their paintings will get detrimental reinforcement, just like the takeaway of a virtual tool.
“It is the similar thought in reinforcement studying,” Chatterjee stated. “The agent can make a choice from a suite of movements. With every motion comes comments, excellent or dangerous, that turns into a part of its reminiscence. There is an interaction between exploring new alternatives and exploiting previous stories. The purpose is to create an agent that learns to make excellent selections.”
Open AI Health club and MITRE ATT&CK
The group used an open-source tool toolkit referred to as Open AI Health club as a foundation to create a customized and regulated simulation atmosphere to judge the strengths and weaknesses of 4 deep reinforcement studying algorithms.
The group used the MITRE ATT&CK framework, evolved through MITRE Corp., and integrated seven techniques and 15 tactics deployed through 3 distinct adversaries. Defenders have been provided with 23 mitigation movements to check out to halt or save you the development of an assault.
Levels of the assault integrated techniques of reconnaissance, execution, endurance, protection evasion, command and regulate, assortment and exfiltration (when information is transferred out of the gadget). An assault used to be recorded as a win for the adversary in the event that they effectively reached the overall exfiltration degree.
“Our algorithms function in a aggressive atmosphere—a competition with an adversary intent on breaching the gadget,” stated Chatterjee. “It is a multistage assault, the place the adversary can pursue more than one assault paths that may exchange through the years as they are attempting to move from reconnaissance to exploitation. Our problem is to turn how defenses in response to deep reinforcement studying can forestall such an assault.”
DQN outpaces different approaches
The group skilled defensive brokers in response to 4 deep reinforcement studying algorithms: DQN (Deep Q-Community) and 3 permutations of what is referred to as the actor-critic manner. The brokers have been skilled with simulated information about cyberattacks, then examined in opposition to assaults that they’d now not noticed in coaching.
DQN carried out the most productive.
- Least refined assaults (in response to various ranges of adversary ability and endurance): DQN stopped 79 % of assaults halfway via assault phases and 93 % through the overall degree.
- Slightly refined assaults: DQN stopped 82 % of assaults halfway and 95 % through the overall degree.
- Maximum refined assaults: DQN stopped 57 % of assaults halfway and 84 % through the overall degree—a long way upper than the opposite 3 algorithms.
“Our purpose is to create an independent protection agent that may be informed the possibly subsequent step of an adversary, plan for it, after which reply in the easiest way to offer protection to the gadget,” Chatterjee stated.
Regardless of the growth, no person is able to entrust cyber protection fully as much as an AI gadget. As an alternative, a DRL-based cybersecurity gadget would want to paintings in live performance with people, stated co-author Arnab Bhattacharya, previously of PNNL.
“AI will also be excellent at protecting in opposition to a particular technique however is not as excellent at working out all of the approaches an adversary may take,” Bhattacharya stated. “We’re nowhere close to the degree the place AI can exchange human cyber analysts. Human comments and steering are vital.”
The analysis is printed at the arXiv preprint server.
Additional information:
Ashutosh Dutta et al, Deep Reinforcement Finding out for Cyber Gadget Protection underneath Dynamic Opposed Uncertainties, arXiv (2023). DOI: 10.48550/arxiv.2302.01595
Quotation:
Cybersecurity defenders are increasing their AI toolbox (2023, February 16)
retrieved 27 February 2023
from https://techxplore.com/information/2023-02-cybersecurity-defenders-ai-toolbox.html
This file is matter to copyright. Aside from any truthful dealing for the aim of personal learn about or analysis, no
section could also be reproduced with out the written permission. The content material is equipped for info functions best.
Supply By way of https://techxplore.com/information/2023-02-cybersecurity-defenders-ai-toolbox.html